25 December 2024
Chatbots. You’ve probably interacted with them more times than you realize. Whether it’s a customer service bot on a website or a friendly assistant like Siri or Alexa, chatbots are becoming a huge part of our digital lives. But have you ever wondered how they work? Or better yet, how you can build one yourself?
In this article, we’re diving into how to build chatbots using Python and Natural Language Processing (NLP). Python is an incredibly versatile programming language and, when paired with NLP, it can be used to create powerful chatbots that understand and respond to human language.
Let’s get ready to roll up our sleeves and get into the nitty-gritty of chatbot creation. Don’t worry; I’ll guide you through the entire process—from understanding the basics to writing your first lines of code.
What is a Chatbot?
Before we get into the technical stuff, let’s make sure we’re on the same page. A chatbot is a program designed to simulate human conversations. It can understand questions, give appropriate responses, and even learn from interactions over time.Think of a chatbot as a digital assistant that can hold a conversation with users. Some are simple, like those FAQ bots that help you find specific information, while others are more advanced, such as virtual assistants like Google Assistant or Microsoft’s Cortana.
The key to making chatbots smarter? You guessed it — Natural Language Processing.
What is Natural Language Processing (NLP)?
Natural Language Processing (NLP) is a field of Artificial Intelligence (AI) that focuses on the interaction between computers and humans using natural language. In simpler terms, NLP helps machines understand and interpret human language in a way that's both meaningful and useful.Without NLP, a chatbot would be nothing more than a glorified keyword search tool. NLP allows chatbots to understand context, sentiment, and even slang, making the interaction more human-like.
Why Python for Chatbots?
There are several reasons why Python is the go-to programming language for building chatbots:- Simplicity: Python’s syntax is simple and easy to read, which makes it an excellent choice for beginners and experienced developers alike.
- Libraries: Python has a ton of libraries that make NLP and chatbot development a breeze (more on that in a bit).
- Community Support: Python has an enormous community, meaning there’s plenty of support, tutorials, and resources available.
Now that we’ve covered the basics, let’s get to the fun part — building a chatbot using Python!
Step-by-Step Guide to Building Your Own Chatbot with Python and NLP
Step 1: Setting Up Your Environment
Before you can start coding, you need to set up your development environment. Here’s what you’ll need:Install Python
If you haven't already, you’ll need to download and install Python. You can grab the latest version of Python from the official Python website.Install Required Libraries
We'll be using a few Python libraries to help us with NLP and chatbot development. Open your terminal and install the following libraries using `pip`:bash
pip install nltk
pip install tensorflow
pip install keras
pip install flask
pip install numpy
pip install scikit-learn
These libraries are essential for NLP tasks, machine learning, and deploying your chatbot.
Step 2: Understanding the Libraries
Let’s take a quick look at what some of these libraries do:- NLTK: The Natural Language Toolkit (NLTK) is a powerful library for processing natural language. It’s the backbone of many NLP tasks such as tokenization, stemming, and sentiment analysis.
- TensorFlow: TensorFlow is a machine learning library that helps train models for tasks like classification and prediction.
- Keras: Keras is a high-level neural networks API that runs on top of TensorFlow, simplifying the creation of deep learning models.
- Flask: Flask is a lightweight web framework. We’ll use it to deploy our chatbot as a web app.
- NumPy: NumPy is useful for handling numerical operations and arrays—key components in machine learning.
- scikit-learn: This library is great for machine learning tasks such as data preprocessing and model evaluation.
Step 3: Preprocessing the Data
To build an intelligent chatbot, we need to train it on a dataset. Let’s assume you have a dataset of questions and answers. Before feeding this data into our model, we need to preprocess it.Tokenization
Tokenization is the process of breaking down text into smaller parts (usually words or phrases). You can think of this as separating a sentence into its individual words.python
import nltk
from nltk.tokenize import word_tokenizenltk.download('punkt')
sample_text = "Hi! How are you doing today?"
tokens = word_tokenize(sample_text)
print(tokens)
This will output:
bash
['Hi', '!', 'How', 'are', 'you', 'doing', 'today', '?']
Stemming
Stemming reduces words to their root form. For example, “running” becomes “run”. This helps the chatbot understand different variations of the same word.python
from nltk.stem import PorterStemmerps = PorterStemmer()
stemmed_words = [ps.stem(w) for w in tokens]
print(stemmed_words)
This will output:
bash
['hi', '!', 'how', 'are', 'you', 'do', 'today', '?']
Step 4: Building the Chatbot Model
Now that our data is preprocessed, it’s time to build the chatbot model. We’ll use a simple neural network model for this.python
import numpy as np
from keras.models import Sequential
from keras.layers import Dense, Activation, Dropout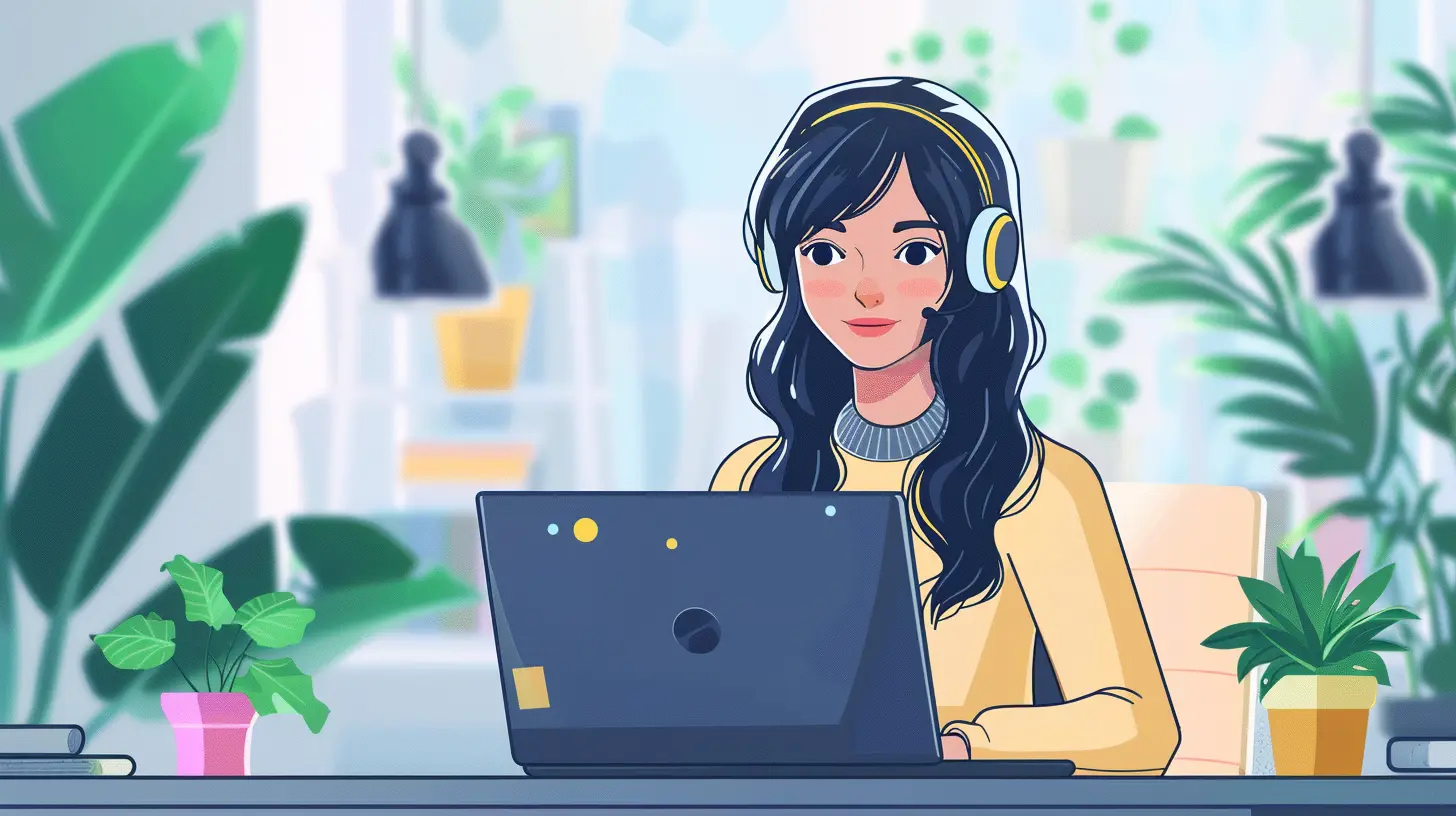
Create a sequential model
model = Sequential()Add layers to the model
model.add(Dense(128, input_shape=(len(train_x[0]),), activation='relu'))
model.add(Dropout(0.5))
model.add(Dense(64, activation='relu'))
model.add(Dropout(0.5))
model.add(Dense(len(train_y[0]), activation='softmax'))Compile the model
model.compile(loss='categorical_crossentropy', optimizer='adam', metrics=['accuracy'])Train the model
model.fit(np.array(train_x), np.array(train_y), epochs=200, batch_size=5, verbose=1)
Here’s what’s happening:
- We’re creating a neural network with three layers.
- The model is compiled using categorical crossentropy as the loss function (since we’re dealing with multiple categories).
- Finally, we train the model on our preprocessed data.
Step 5: Building the Response Function
Once your chatbot can recognize different inputs, you’ll want it to respond accordingly. Let’s create a simple function that looks for a match in the model’s predictions and returns an appropriate response.python
import randomdef get_response(intents_list, intent_json):
tag = intents_list[0]['intent']
list_of_intents = intent_json['intents']
for i in list_of_intents:
if i['tag'] == tag:
result = random.choice(i['responses'])
break
return result
Step 6: Deploying the Chatbot with Flask
Now that we have a working chatbot, the final step is to deploy it as a web app using Flask. Here’s a basic setup:python
from flask import Flask, render_template, request
app = Flask(__name__)@app.route("/")
def home():
return render_template("index.html")
@app.route("/get")
def get_bot_response():
userText = request.args.get('msg')
return str(chatbot_response(userText))
if __name__ == "__main__":
app.run()
In this example, the `/get` route handles user input and returns a response from the chatbot. You can extend this to create a more interactive web interface.
Step 7: Testing and Improving Your Chatbot
Once deployed, you can start testing your chatbot. Don’t expect it to be perfect right away. Chatbot development is an iterative process, and you’ll need to keep tweaking the bot’s training data, model, and responses to improve its accuracy.Wrapping Up
And there you have it! You’ve just built a basic chatbot using Python and NLP. While this example is relatively simple, you can make your chatbot more sophisticated by adding features like sentiment analysis, context retention, or even voice recognition.The possibilities are endless, and with Python’s vast array of libraries and tools, the only limit is your imagination.
So, what kind of chatbot are you going to build next? Maybe a virtual shopping assistant, a personal finance manager, or even a language learning buddy? The choice is yours.
Happy coding!
Thorne Clarke
This article provides a solid foundation for anyone looking to create chatbots using Python and NLP. The step-by-step approach is clear and practical, making complex concepts accessible. If you're interested in AI development, this is a great starting point. Looking forward to seeing more tutorials like this!
January 28, 2025 at 9:42 PM